Accelerating therapeutic innovations by harnessing generative AI for smarter clinical trials
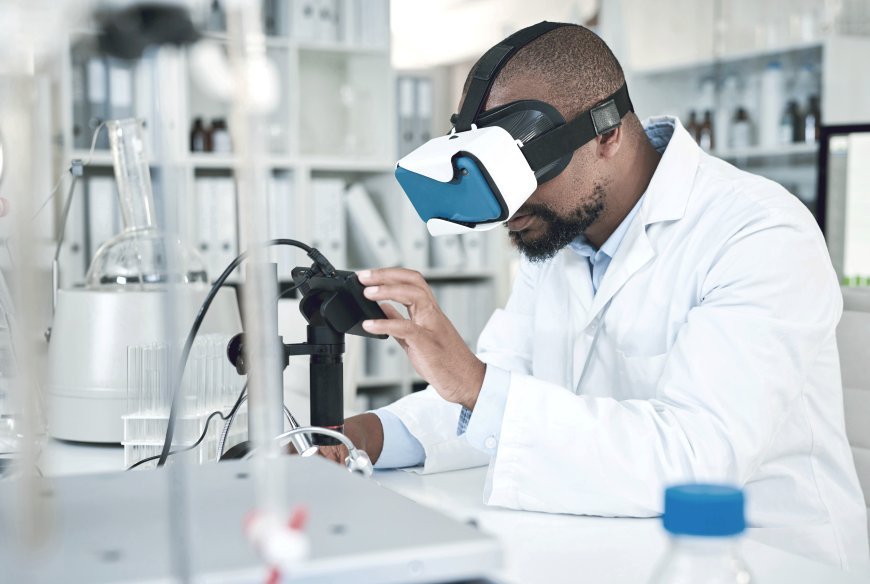
Stakeholders in life sciences have struggled to accelerate drug development while reducing costs, with inefficiencies in clinical development being a major barrier. Despite significant investment, the challenges have only grown. Even with various strategies by healthcare consulting firms to reduce failures, the success rate for clinical drug development remains low. With generative AI (gen AI) the pharma industry can transform this landscape by significantly reducing development time and costs. Here are five key processes in clinical development that are primed for transformation and offer the potential to reduce costs, shorten timelines and improve success rates:
The trial design Challenges: Clinical trial protocols define key study elements, including objectives, methodology, inclusion criteria and execution plans. These protocols are critical in determining a trial’s success, yet the design process remains highly complex and inefficient. Manual workflows, reliance on historical data and subjective decision-making often result in suboptimal trial designs. This further leads to long development cycles, high costs and increased failure rates.
Future: Artificial intelligence in pharma has the potential to revolutionize trial design by leveraging unstructured data from past protocols, real-world data (RWD), regulatory guidelines and patient feedback. AI-driven systems can assist in developing trial concepts, optimizing statistical models, refining protocols and simulating various scenarios to improve decision-making.
Over time, Gen AI will enable innovations such as digital and surrogate endpoints, synthetic control arms and fully simulated in silico trials. By automating manual processes, AI will enhance predictive accuracy, reduce errors, eliminate redundant work and streamline protocol development. This will lower costs, minimize administrative burdens and accelerate trial initiation.
Trial feasibility and site selection
Challenges: The increasing complexity of clinical trials has made assessing feasibility and selecting sites more challenging. Patient enrollment, a critical factor in trial success, often takes 1.8 times longer than expected, with 85% of trials experiencing shortfalls.
Currently, site selection relies on historical data and past trial experiences, using basic metrics like site capacity and previous enrollment rates. However, this approach overlooks more relevant factors such as local patient demographics, disease prevalence and site-specific capabilities. The lack of comprehensive site profiles, siloed data and limited real-time insights leads to under-enrollment, delays and poor feasibility assessments.
Future: Generative AI can transform site selection by analyzing unstructured data from past trials and real-time real-world data (RWD) to predict trial outcomes more accurately. By integrating gen AI with predictive AI, trial planners can better assess a site's recruitment potential, identify optimal locations and anticipate enrollment challenges before they arise.
Additionally, AI-driven simulations can model different site configurations to determine the best strategies for patient recruitment and retention. Gen AI can also support decentralized trials by managing logistics across multiple sites and investigators, making clinical trials more efficient, patient-centric and adaptable to real-world conditions.
Clinical operations
Challenges: Patient recruitment in clinical trials relies on traditional methods like physician referrals, patient registries and site-driven outreach. These are time-consuming and often ineffective in reaching diverse populations. Retention strategies, such as periodic check-ins and incentives, frequently fall short, leading to high dropout rates. While digital endpoints help reduce participant burden, challenges like poor communication and logistical difficulties with site visits continue to impact retention.
Engagement remains reactive and one-size-fits-all, with limited use of predictive tools to identify at-risk participants or personalize retention efforts. Additionally, site burden remains a significant challenge, further complicating trial efficiency.
Future: With gen AI, the pharma industry can transform clinical operations by analyzing data from various sources, including electronic health records (EHRs), insurance claims and patient advocacy groups. This data can fuel predictive models that identify potential dropouts and recommend personalized engagement strategies to improve participant retention. Essentially, AI will enable tailored “marketing plans” to enhance the patient experience, ensuring better enrollment, sustained engagement and overall trial success.
Clinical trial data analysis
Challenges: As data collection methods become more advanced, clinical trial teams face increasing difficulties in ensuring data quality and standardization. Data analysis remains a time-intensive process, requiring specialized expertise and extensive manual effort. Analysts must work with fragmented data sources such as electronic health records (EHRs), lab results and patient-reported outcomes, which often lack seamless integration.
Before analysis can begin, significant time is spent on data cleaning, transformation and validation. Additionally, coding tasks for data processing and statistical analysis are still partially manual, making them prone to human error, costly and slow.
Future: Clinical trial data analysis, assisted by generative AI, can act as a co-pilot that can automate repetitive tasks such as writing code and generating submission-ready tables. It can also streamline data integration by quickly processing diverse sources, including patient engagement data from chatbots, mobile apps and wearable sensors. By automating data preparation and applying predictive AI methods, gen AI can significantly reduce the time between data collection and actionable insights, accelerating decision-making and improving trial efficiency.
Regulatory submission
Challenges: The growing complexity of clinical trials has led to inefficiencies in regulatory submissions, with the number of procedures per trial increasing by 60% over the past decade. Submission timelines have also lengthened, and many companies still rely on manual processes, resulting in frequent errors. Additionally, regulatory teams often work in silos, leading to inconsistencies and duplicated efforts.
Future: Harnessing generative AI can streamline regulatory submissions by automating tasks such as generating, organizing and validating filings. AI-driven tools will compile trial data into required formats, cross-reference information, flag omissions and ensure compliance. This reduces costly delays caused by requests for additional information. Furthermore, gen AI can support predictive algorithms to assess the likelihood of regulatory approval, helping companies optimize their submission strategies.
With the right investments and regulatory support, gen AI can optimize trial design, improve site selection, streamline clinical operations, automate data analysis and accelerate regulatory submissions. Beyond improving traditional trials, AI-driven approaches could enable adaptive trial designs and continuous learning based on real-time RWE. However, several challenges must be addressed, including fragmented data systems, lack of standardized infrastructure, limited incentives for data sharing, regulatory uncertainties and skill gaps. In addition, stakeholders should aim to collaborate as the stakes are about affordability, effective clinical trials and getting access to high-quality treatments.
About the Author:
The author writes healthcare journals and articles, having keen interest and expertise in this industry. She has extensive experience in working with a leading healthcare consultancy.
What's Your Reaction?
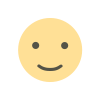
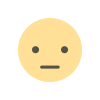
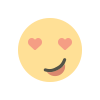

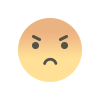
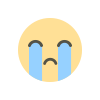
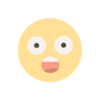